As the amount of data available to law enforcement agencies increases, so does the pressure for them to use that data in efficient and predictive ways to thwart crime. Centrality analysis helps agencies ask specific questions of their big data and get answers quickly.
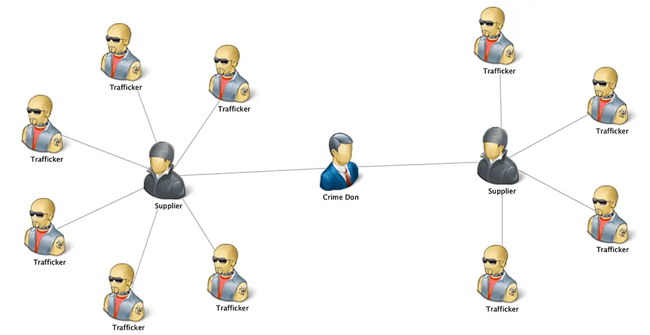
We’ve applied our graph analytics and visualization expertise to tough problems in law enforcement, fraud detection, cybersecurity, telecommunications, and more. Of the 30+ data analysis algorithms available in Tom Sawyer Perspectives, there are four centrality algorithms that answer questions like:
- Who has the most direct connections?
- Who are the top-tier influencers?
- Who are the middlemen?
- Who is controlling the information flow?
Because there is no one-size-fits-all method for analyzing centrality, we’ll use a different algorithm to answer each question. To visualize the results, we used a sample graph analysis project included in Tom Sawyer Perspectives. It uses fabricated data about the network of crime boss Narco Polo.
Degree Centrality Analysis
Degree Centrality analysis identifies the individuals with the most direct connections, or the node with the most edges, in a network. Simply put, this analysis shows the most popular people. Alternatively, if the node represents a crime, Degree Centrality highlights which crime involved the most people.
It’s easy: a node with 15 edges has a higher Degree Centrality than a node with 8 edges.
The Visualization
You might assume that the crime boss himself is the most popular individual in his own network. However, Degree Centrality analysis reveals that dealer Connie Jewel is the most connected player. She has 16 direct connections while Narco Polo only has 4.
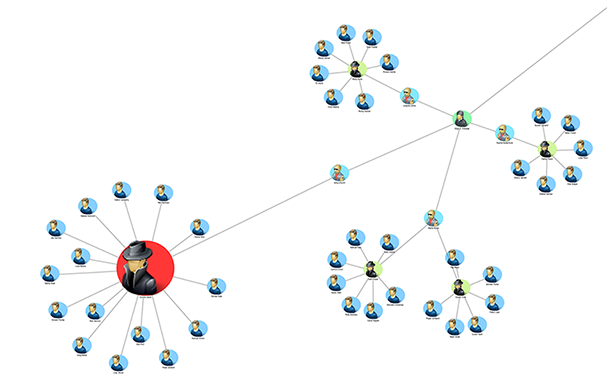
Closeness Centrality Analysis
Closeness Centrality analysis determines the importance of each individual based on how many middlemen are required to reach all the other individuals in the network. This analysis looks further out than the direct connections of Degree Centrality to instead discover who is closest to all the people in a network.
Similar to the “six degrees of separation” theory, this analysis method highlights nodes that have the smallest average distance to all other nodes in the network. In this case, distance is the number of hops or edges that are required to get from one node to another.
The Visualization
It’s not surprising that Narco Polo has the highest Closeness Centrality ranking. However, this visualization also shows the four suppliers as important middlemen in the network.
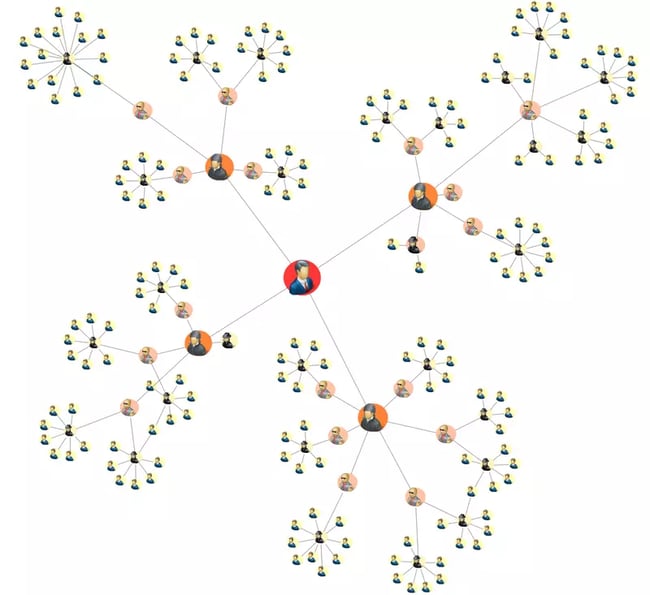
Betweenness Centrality Analysis
Betweenness Centrality analysis highlights the individuals closest to a high flow of activity. By identifying a person with a high Betweenness Centrality, you can see who controls the flow of information in the network.
To analyze the flow, this algorithm examines paths. The Shortest Path shows the smallest number of hops or edges between two nodes. The All Pairs Shortest Path shows all of the shortest paths in the network between each pair of nodes. That’s a lot of paths!
Think of these paths as superhighways. Nodes that are part of many superhighways could be worth investigating. It’s likely that lots of information, goods, and activities flow through them. Further, these nodes could be particularly interesting to disrupt. Arresting the most effective criminal could slow down the activity of the entire crime network.
The Visualization
As expected, Narco Polo ranks highest for Betweenness Centrality in his own network; removing him would result in the network’s collapse. But who comes in second? Here, our visualization shows supplier Jane F. Sample as the most important intermediary.
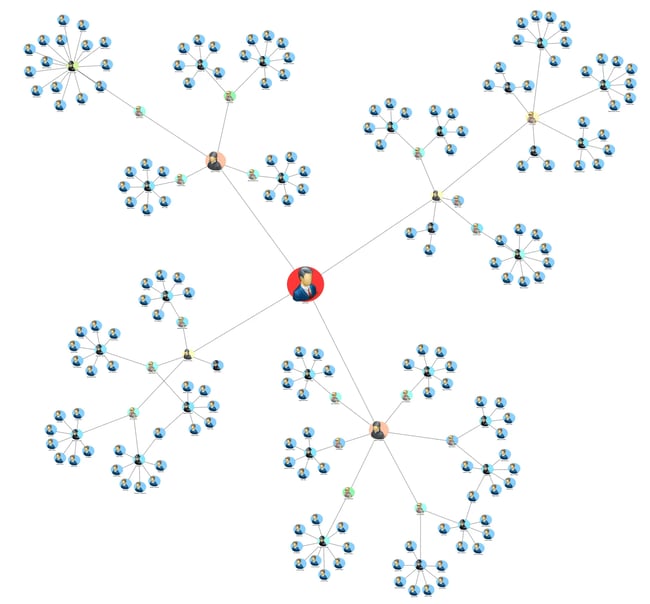
Eigenvector Centrality Analysis
So far, each algorithm we’ve covered gives all connections equal weight. For example, if we were examining an organizational network, Degree Centrality, Closeness Centrality, and Betweenness Centrality would weigh connections among peers the same as connections to executive leadership. But in reality, all connections are not equal.
Understanding that some connections are more influential than others, Eigenvector Centrality analysis determines the importance of each node based on the importance of its connections. Thus, nodes will become more central by the power of their associations—not simply by the volume of their connections. Visit Geeks for Geeks for more on the math behind this analysis.
The Visualization
According to our Degree Centrality analysis, Connie Jewel boasts the most direct connections in the network. However, upon closer examination, most of her connections are end-of-the-line users. Her connections aren’t very important. Instead, Eigenvector Centrality analysis shows that trafficker Leo Smith controls much of the information flow in this crime network. Leo connects to the crime boss and five higher level dealers, not just to users.
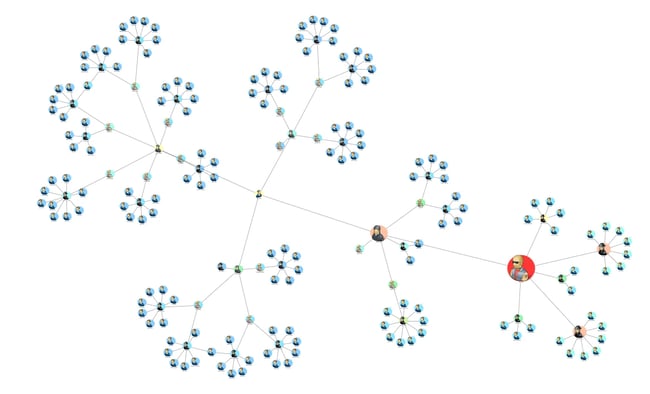
Perspectives Pro Tip
For some investigations, the answer to one of our analysis questions isn’t enough. Tom Sawyer Perspectives to the rescue! After running through each of the centralities, the Chart View shows the results of all the analyses so you can compare the players at a glance. For our sample case, we can see that Narco Polo is very high in Betweenness Centrality but much lower in the other analyses. However, supplier Jane F. Sample ranks relatively high across all of the analysis methods.
This feature allows law enforcement to get the most from their data. By knowing the goal of a specific analysis, they can use the best centrality method—or perhaps a combination of all of them.
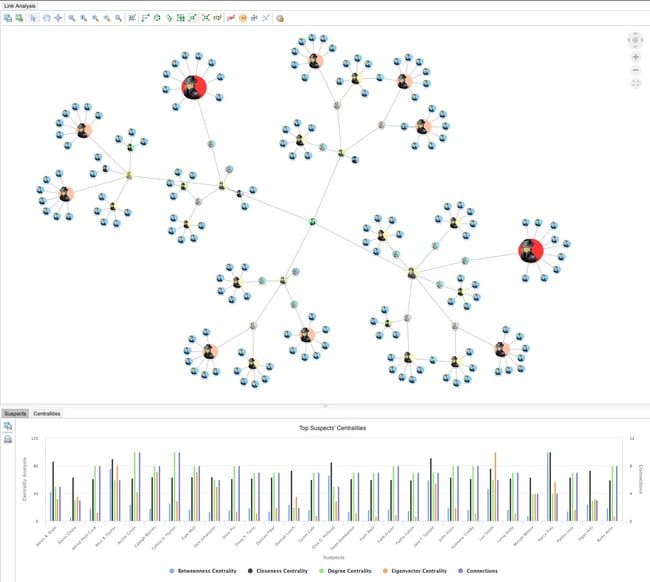
To perform centrality analysis in Perspectives, try the Crime Network example application for free today. You can also try the Panama Papers example application to see how the same centrality analysis methods provide insight into knowledge networks and organizational networks.
About the Author
Kristin Petersen is the Marketing Content Manager at Tom Sawyer Software. She has 17+ years of technical writing and editing experience in both the public and private sector, and transitioned into Marketing where she brings her strong technical background, writing experience, and creativity. She earned her Master of Science in Technical Communication from Drexel.
Submit a Comment